Baltasar Beferull-Lozano
ProfessorChief Research Scientist/Research ProfessorHead of Department
- Department
- Signal and Information Processing for Intelligent Systems
- Organisation
- Simula Metropolitan
- Research Interests
- data science and machine learning online optimization graph signal processing intelligent sensing and signal processing cyber-physical systems in-network distributed and cooperative intelligence AI-driven networks and systems
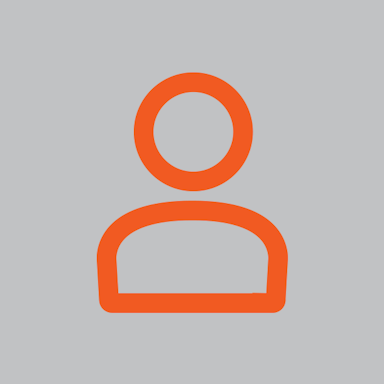
- baltasar@simula.no
Publications
2024
Journal Articles
R. K. Jaiswal, M. Elnourani, S. Deshmukh and B. Beferull-Lozano
A Data-driven Transfer Learning Method for Indoor Radio Map Estimation
IEEE Transactions on Wireless Communications
J. Krishnan, R. Money, B. Beferull-Lozano and E. Isufi
Simplicial Vector Autoregressive Models
IEEE Transactions on Signal Processing
Conference proceedings
S. Dominguez-Cid, J. Krishnan, R. Money, B. Beferull-Lozano, D. F. Larios-Marin and C. L. de Mora
Graph-Vector Auto-regressive Model for Lithium-Ion Cell Capacity Estimation
IEEE Machine Learning for Signal Processing
J. Singh, J. Zhou, B. Beferull-Lozano, S. Yan and S. Ghori
Haptic Teleoperation Framework for Learning Task Space Fine Manipulation Skills
IEEE ICIEA
R. Money, J. Krishnan and B. Beferull-Lozano
Limited Data Forecasting of Financial Time-series using Graph-based Class Dynamics
EUSIPCO
R. Money, J. Krishnan, B. Beferull-Lozano and E. Isufi
Evolution Backcasting of Edge Flows from Partial Observations using Simplicial Vector Autoregressive Models
The 49th IEEE International Conference on Acoustics, Speech, & Signal Processing (ICASSP)
2023
Journal Articles
E. Ruiz and B. Beferull-Lozano
An Online Multiple Kernel Parallelizable Learning Scheme
IEEE Signal Processing Letters
B. Zaman, L. M. Lopez-Ramos and B. Beferull-Lozano
Online Joint Topology Identification and Signal Estimation from Streams with Missing Data
IEEE Transactions on Signal and Information Processing over Networks
K. Roy, L. M. Lopez-Ramos and B. Beferull-Lozano
Efficient Interpretable Nonlinear Modeling for Multiple Time Series
IEEE Transactions on Signal Processing
R. Money, J. Krishnan and B. Beferull-Lozano
Sparse Online Learning with Kernels using Random Features for Estimating Nonlinear Dynamic Graphs
IEEE Transactions on Signal Processing
E. Ruiz, L. M. Lopez-Ramos and B. Beferull-Lozano
Zero-delay Consistent Signal Reconstruction from Streamed Multi-variate time-series
IEEE Transactions on Signal Processing
S. Mollaebrahim and B. Beferull-Lozano
Distributed Linear Network Operators Based on Successive Graph Shift Matrices
IEEE Transactions on Signal and Information Processing over Networks
E. Ruiz, L. M. Lopez-Ramos and B. Beferull-Lozano
A Trainable Approach to Zero-delay Smoothing Spline Interpolation
IEEE Transactions on Signal Processing
Proceedings, refereed
A. Nama, L. Ben Saad, B. Beferull-Lozano and J. Zhou
Neighborhood Graph Filters based Graph Convolutional Neural Networks for Multi-Agent Deep Reinforcement Learning
IECON - Annual Conference of the IEEE Industrial Electronics Society
Conference proceedings
J. Singh, J. Zhou and B. Beferull-Lozano
Enhancing Multi-Agent Reinforcement Learning: Set Function Approximation and Dynamic Policy Adaptation
IECON - Annual Conference of the IEEE Industrial Electronics Society
2022
Journal Articles
R. Money, J. Krishnan, B. Beferull-Lozano and E. Isufi
Online Edge Flow Imputation on Networks
IEEE Signal Processing Letters
R. Money, J. Krishnan, B. Beferull-Lozano and E. Isufi
Scalable and Privacy-aware Online Learning of Nonlinear Structural Equation Models
IEEE Open Journal of Signal Processing
M. Elnourani, S. Deskmukh and B. Beferull-Lozano
Resource Allocation for Underlay Interfering D2D Networks with Multi-antenna and Imperfect CSI
IEEE Transactions on Communications
M. Elnourani, S. Deshmukh, B. Beferull-Lozano and D. Romero
Robust Sum-Rate Maximization for Underlay Device-to-Device Communications on Multiple Channels
IEEE Transactions on Vehicular Technology
Proceedings, refereed
R. Money, J. Krishnan and B. Beferull-Lozano
Online Joint Nonlinear Topology Identification and Missing Data Imputation over Dynamic Graphs
30th European Signal Processing Conference (EUSIPCO)
K. Roy, L. M. Lopez-Ramos and B. Beferull-Lozano
Joint Learning of Topology and Invertible Nonlinearities from Multiple Time Series
IEEE International Conference on Machine Learning, Optimization, and Data Science (ISMODE)
R. Money, J. Krishnan and B. Beferull-Lozano
Online Joint Nonlinear Topology Identification and Missing Data Imputation over Dynamic Graphs
30th European Signal Processing Conference (EUSIPCO)
L. Ben Saad, A. N. Nama and B. Beferull-Lozano
Neighborhood Graph Neural Networks under Random Perturbations and Quantization Errors
IEEE International Conference on Signal Processing Advances in Wireless Communications (SPAWC)
R. K. Jaiswal, M. Elnourani, S. Deshmukh and B. Beferull-Lozano
Deep Transfer Learning Based Radio Map Estimation for Indoor Wireless Communications
IEEE International Conference on Signal Processing Advances in Wireless Communications (SPAWC)
K. Roy, L. M. Lopez-Ramos and B. Beferull-Lozano
Joint Signal Estimation and Nonlinear Topology Identification from Noisy Data with Missing Entries
IEEE Asilomar Conference on Signals, Systems, and Computers
J. D. Cardenas-Cartagena, M. Elnourani, B. Beferull-Lozano and D. Romero
Risk-Aware Particle-Filtering for State Estimation in Recirculating Aquaculture Systems
IEEE Asilomar Conference on Signals, Systems, and Computers
R. K. Jaiswal, S. Deshmukh, M. Elnourani and B. Beferull-Lozano
Transfer Learning Based Joint Resource Allocation for Underlay D2D Communications
IEEE Wireless Communications and Networking Conference (WCNC)
2021
Journal Articles
J. Bhardwaj, J. Krishnan, D. F. L. Marin, B. Beferull-Lozano, L. R. Cenkeramaddi and C. Harman
Cyber-Physical Systems for Smart Water Networks: A Review
IEEE Sensors Journal
L. Ben Saad, B. Beferull-Lozano and E. Isufi
Quantization Analysis and Robust Design in Distributed Graph Filters
IEEE Transactions on Signal Processing
R. Sharan, S. Deshmukh, S. R. B. Pillai and B. Beferull-Lozano
Energy Efficient AoI Minimization in Opportunistic NOMA/OMA Broadcast Wireless Networks
IEEE Transactions on Green Communications and Networking
Proceedings, refereed
R. Money, J. Krishnan and B. Beferull-Lozano
Online Non-linear Topology Identification from Graph-connected Time Series
2021 IEEE Data Science and Learning Workshop (DSLW)2021 IEEE Data Science and Learning Workshop (DSLW)
J. Bhardwaj, J. Krishnan and B. Beferull-Lozano
Data-Driven Pump Scheduling for Cost Minimization in Water Networks
2021 IEEE International Conference on Autonomous Systems (ICAS)2021 IEEE International Conference on Autonomous Systems (ICAS)
R. Money, J. Krishnan and B. Beferull-Lozano
Random Feature Approximation for Online Nonlinear Graph Topology Identification
2021 IEEE 31st International Workshop on Machine Learning for Signal Processing (MLSP) 2021 IEEE 31st International Workshop on Machine Learning for Signal Processing (MLSP)
E. Ruiz and B. Beferull-Lozano
Tracking of quantized signals based on online kernel regression
IEEE International Workshop on Machine Learning for Signal Processing (MLSP)
R. Money, J. Krishnan and B. Beferull-Lozano
Online Non-linear Topology Identification from Graph-connected Time Series
2021 IEEE Data Science and Learning Workshop (DSLW)2021 IEEE Data Science and Learning Workshop (DSLW)
J. Bhardwaj, J. Krishnan and B. Beferull-Lozano
Data-Driven Pump Scheduling for Cost Minimization in Water Networks
2021 IEEE International Conference on Autonomous Systems (ICAS)2021 IEEE International Conference on Autonomous Systems (ICAS)
R. Money, J. Krishnan and B. Beferull-Lozano
Random Feature Approximation for Online Nonlinear Graph Topology Identification
2021 IEEE 31st International Workshop on Machine Learning for Signal Processing (MLSP) 2021 IEEE 31st International Workshop on Machine Learning for Signal Processing (MLSP)