Carla Schenker
Postdoctoral Fellow
- Department
- Data Science and Knowledge Discovery
- Organisation
- Simula Metropolitan
- Research Interests
- Matrix and Tensor Factorizations
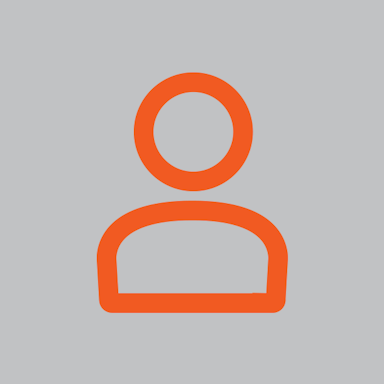
- carla@simula.no
Publications
2024
Journal Articles
C. Chatzis, C. Schenker, M. Pfeffer and E. A. Ataman
tPARAFAC2: Tracking evolving patterns in (incomplete) temporal data
arXiv Preprints
Talks, invited
C. Chatzis, C. Schenker, M. Pfeffer, P. Lind and E. A. Ataman
A Time-aware tensor decomposition for concept evolution
94th Annual meeting of the International Association of Applied Mathematics and Mechanics (GAMM 2024)
Miscellaneous
C. Schenker, X. Wang, D. Horner, M. A. Rasmussen and E. A. Ataman
PARAFAC2-based Coupled Matrix and Tensor Factorizations with Constraints
2023
Proceedings, refereed
C. Schenker, X. Wang and E. A. Ataman
PARAFAC2-based coupled Matrix and Tensor Factorizations
IEEE International Conference on Acoustics, Speech and Signal Processing (ICASSP)
PhD Thesis
C. Schenker
A Flexible Framework for Data Fusion Based on Coupled Matrix and Tensor Factorizations for Interpretable Pattern Discovery
Oslo Metropolitan University, Norway
Posters
C. Schenker, X. Wang and E. A. Ataman
PARAFAC2-Based Coupled Matrix and Tensor Factorizations
IEEE International Conference on Acoustics, Speech and Signal Processing (ICASSP)
Talks, invited
C. Schenker, X. Wang and E. A. Ataman
PARAFAC2-Based Coupled Matrix and Tensor Factorizations with Constraints
10th International Congress on Industrial and Applied Mathematics, ICIAM 2023, Tokyo, Japan
C. Schenker, X. Wang and E. A. Ataman
Fusing Dynamic and Static Data Using Parafac2 Based Coupled Matrix and Tensor Factorizations
SIAM Conference on Computational Science and Engineering
2022
Journal Articles
M. Roald, C. Schenker, V. D. Calhoun, T. Adali, R. Bro, J. E. Cohen and E. A. Ataman
An AO-ADMM approach to constraining PARAFAC2 on all modes
SIAM Journal on Mathematics of Data Science
Talks, invited
C. Schenker, M. Roald, X. Wang, J. E. Cohen and E. A. Ataman
Constrained Multi-Modal Data Mining Using Coupled Matrix and Tensor Factorizations
SIAM Conference on Mathematics of Data Science
Talks, contributed
M. Roald, C. Schenker, V. D. Calhoun, T. Adali, R. Bro, J. E. Cohen and E. A. Ataman
An AO-ADMM approach to constrained PARAFAC2
Nordic AI Meet
M. Roald, C. Schenker, R. Bro, J. E. Cohen and E. A. Ataman
Fully Constrained PARAFAC2 with AO-ADMM
SIAM Conference on Parallel Processing for Scientific Computing
2021
Journal Articles
C. Schenker, J. E. Cohen and E. A. Ataman
A Flexible Optimization Framework for Regularized Matrix-Tensor Factorizations with Linear Couplings
IEEE Journal of Selected Topics in Signal Processing
Proceedings, refereed
M. Roald, C. Schenker, J. E. Cohen and E. A. Ataman
PARAFAC2 AO-ADMM: Constraints in all modes
2021 29th European Signal Processing Conference (EUSIPCO)
Posters
C. Schenker, J. E. Cohen and E. A. Ataman
An Optimization Framework for Regularized Linearly Coupled Matrix-Tensor Factorization
Talks, invited
C. Schenker, J. E. Cohen and E. A. Ataman
A Flexible Optimization Framework for Regularized Linearly Coupled Matrix-Tensor Factorizations based on the Alternating Direction Method of Multipliers
Europt21, 18th Workshop on Advances in Continuous Optimization
C. Schenker, J. Cohen and E. A. Ataman
An Optimization Framework for Regularized Linearly Coupled Matrix-Tensor Factorization
SIAM Conference on Applied Linear Algebra (LA21)
Talks, contributed
C. Schenker, M. Roald, J. E. Cohen and E. A. Ataman
A Flexible Optimization Framework for Regularized Matrix-Tensor Factorizations with Linear Couplings
Asilomar Conference on Signals, Systems, and Computers
M. Roald, C. Schenker, J. E. Cohen and E. A. Ataman
Tracing Dynamic Networks through Constrained Parafac2 Decomposition
SIAM Conference on Applied Linear Algebra (LA21), Virtual Conference
2020
Proceedings, refereed
C. Schenker, J. E. Cohen and E. A. Ataman
An Optimization Framework for RegularizedLinearly Coupled Matrix-Tensor Factorization
European Signal Processing Conference (EUSIPCO)
2019
Journal Articles
E. A. Ataman, C. Schenker, Y. Levin-Schwartz, V. D. Calhoun and T. Adali
Unraveling Diagnostic Biomarkers of Schizophrenia Through Structure-Revealing Fusion of Multi-Modal Neuroimaging Data
Frontiers in Neuroscience
Talks, invited
E. A. Ataman, C. Schenker, Y. Levin-Schwartz, V. D. Calhoun and T. Adali
Biomarker Discovery through Structure-Revealing Fusion of Multi-Modal Neuroimaging Data
IEEE EMBC (Engineering in Medicine and Biology Conference), Berlin, Germany