Hugo Hammer
Adjunct Chief Research Scientist
- Department
- Holistic Systems
- Organisation
- Simula Metropolitan
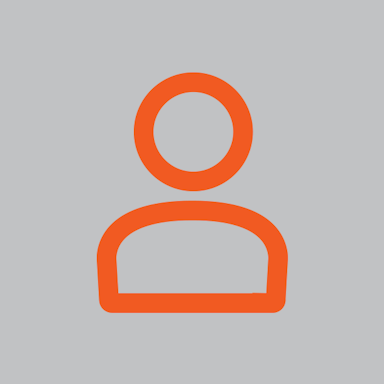
- hugo@simula.no
Publications
2024
Journal Articles
H. Svennevik, S. Hicks, M. Riegler, T. Storelvmo and H. L. Hammer
A dataset for predicting cloud cover over Europe
Scientific Data
M. Vo, T. Nguyen, M. Riegler and H. L. Hammer
Efficient Estimation of Generative Models Using Tukey Depth
Algorithms
Proceedings, refereed
A. Sharma, F. Alawad, R. Kakulavarapu, M. Iliceto, M. Riegler, M. Stensen and H. L. Hammer
Exploring Embryo Development at the Morula Stage-an AI-based Approach to Determine Whether to Use or Discard an Embryo
2024 4th International Conference on Applied Artificial Intelligence (ICAPAI)
2023
Journal Articles
A. Sharma, A. Z. Ansari, K. Radhika, M. Stensen, M. Riegler and H. L. Hammer
Predicting Cell Cleavage Timings from Time-Lapse Videos of Human Embryos
Big Data and Cognitive Computing
M. Q. Vo, T. Nguyen, M. Riegler and H. L. Hammer
Efficient Estimation of Generative Models using Tukey Depth
NA
M. Boeker, P. Jakobsen, M. Riegler, L. Stabell, O. B. Fasmer, P. Halvorsen and H. L. Hammer
Affect Recognition in Muscular Response Signals
IEEE Access
H. H. Thunold and H. L. Hammer
A Deep Diagnostic Framework Using Explainable Artificial Intelligence and Clustering
Diagnostics
V. Thambawita, S. Hicks, A. Storås, T. Nguyen, J. M. Andersen, O. Witczak, T. B. Haugen, H. L. Hammer, P. Halvorsen and M. Riegler
VISEM-Tracking, a human spermatozoa tracking dataset
Scientific Data
H. L. Hammer, M. Riegler and H. Tjelmeland
Approximate Bayesian Inference Based on Expected Evaluation
Bayesian Analysis
Proceedings, refereed
T. Nguyen, H. T. Ly, M. Riegler, P. Halvorsen and H. L. Hammer
Principal Components Analysis based frameworks for efficient missing data imputation algorithms
Recent Challenges in Intelligent Information and Database Systems. ACIIDS 2023
F. Fineide, A. Storås, M. Magnø, H. L. Hammer, X. Chen, A. Khan, P. Halvorsen, T. Utheim and M. Riegler
Predicting the degree of meibomian gland dropout with artificial intelligence
Investigative Ophthalmology & Visual Science, ARVO Annual Meeting
A. Storås, F. Fineide, H. L. Hammer, A. Khan, M. Magnø, X. Chen, P. Halvorsen, T. Utheim and M. Riegler
Using explainable artificial intelligence (XAI) to explore factors affecting meibomian gland (MG) dropout
Investigative Ophthalmology & Visual Science, ARVO Annual Meeting
A. Sharma, M. Stensen, E. Delbarre, M. Siddiqui, T. Haugen, M. Riegler and H. L. Hammer
Detecting human embryo cleavage stages using YOLO v5 object detection algorithm
Nordic Artificial Intelligence Research and Development
2022
Journal Articles
A. Sharma, R. Kakulavarapu, V. Thambawita, M. Siddiqui, E. Delbarre, M. Riegler, H. L. Hammer and M. Stensen
Automating tracking of cell division for human embryo development in time lapse videos
Human Reproduction
V. Thambawita, S. Hicks, A. Storås, O. Witczak, J. M. Andersen, H. L. Hammer, P. Halvorsen, M. Riegler and H. B. Trine
Real-time deep learning based multi object tracking of spermatozoa in fresh samples
Human Reproduction
S. Hicks, V. Thambawita, A. Storås, T. B. Haugen, H. L. Hammer, P. Halvorsen, M. Riegler and M. H. Stensen
Automatic Tracking of the ICSI procedure using Deep Learning
Human Reproduction
M. Boeker, M. Riegler, H. L. Hammer, P. Jakobsen and P. Halvorsen
Prediction of Schizophrenia from Activity Data using Hidden Markov Model Parameters
Neural Computing and Applications
H. L. Hammer, A. Yazidi, M. Riegler and H. Rue
Efficient quantile tracking using an oracle
Applied Intelligence
V. Thambawita, P. Salehi, S. A. Sheshkal, S. Hicks, H. L. Hammer, S. Parasa, T. de Lange, P. Halvorsen and M. Riegler
SinGAN-Seg: Synthetic training data generation for medical image segmentation
PLOS ONE
A. Storås, R. Prabhu, H. L. Hammer and I. Strümke
Bias og kvantitativ analyse innen velferd
Tidsskrift for velferdsforskning
S. S. Eide, M. Riegler, H. L. Hammer and J. B. Bremnes
Deep Tower Networks for Efficient Temperature Forecasting from Multiple Data Sources
Sensors
H. L. Hammer, A. Yazidi and H. Rue
Estimating tukey depth using incremental quantile estimators
Pattern Recognition
A. Storås, I. Strümke, M. Riegler, J. Grauslund, H. L. Hammer, A. Yazidi, P. Halvorsen, K. G. Gundersen, T. P. Utheim and C. J. Jackson
Artificial intelligence in dry eye disease
The Ocular Surface
Proceedings, refereed
V. Thambawita, S. Hicks, A. Storås, J. M. Andersen, O. Witczak, T. B. Haugen, H. L. Hammer, T. Nguyen, P. Halvorsen and M. Riegler
Medico Multimedia Task at MediaEval 2022: Transparent Tracking of Spermatozoa
MediaEval 2022
A. Sharma, R. Kakulavarapu, V. Thambawita, M. Siddiqui, E. Delbarre, M. Riegler, H. L. Hammer and M. Stensen
Automating tracking of cell division for human embryo development in time lapse videos
Human Reproduction
A. Sharma, M. H. Stensen, E. Delbarre, T. B. Haugen and H. L. Hammer
Explainable Artificial Intelligence for Human Embryo Cell Cleavage Stages Analysis
Proceedings of the 3rd ACM Workshop on Intelligent Cross-Data Analysis and Retrieval
A. Storås, M. Riegler, T. B. Haugen, V. Thambawita, S. Hicks, H. L. Hammer, R. Kakulavarapu, P. Halvorsen and M. Stensen
Automatic Unsupervised Clustering of Videos of the Intracytoplasmic Sperm Injection (ICSI) Procedure
NAIS: Symposium of the Norwegian AI Society
2021
Journal Articles
A. Sharma, T. Haugen, H. L. Hammer, M. Riegler and M. Stensen
Towards better explainable deep learning models for embryo selection in ART
Human Reproduction
V. Thambawita, J. L. Isaksen, S. Hicks, J. Ghouse, G. Ahlberg, A. Linneberg, N. Grarup, C. Ellervik, M. S. Olesen, T. Hansen, C. Graff, N. Holstein-Rathlou, I. Strümke, H. L. Hammer, M. Maleckar, P. Halvorsen, M. Riegler and J. K. Kanters
DeepFake electrocardiograms using generative adversarial networks are the beginning of the end for privacy issues in medicine
Nature Scientific Reports
H. Svennevik, M. Riegler, S. Hicks, T. Storelvmo and H. L. Hammer
Prediction of Cloud Fractional Cover Using Machine Learning
Big Data and Cognitive Computing
V. Thambawita, J. L. Isaksen, S. Hicks, J. Ghouse, G. Ahlberg, A. Linneberg, N. Grarup, C. Ellervik, M. S. Olesen, T. Hansen, C. Graff, N. Holstein-Rathlou, I. Strümke, H. L. Hammer, M. Maleckar, P. Halvorsen, M. Riegler and J. K. Kanters
DeepFake electrocardiograms: the key for open science for artificial intelligence in medicine
Scientific Reports
M. Riegler, M. H. Stensen, O. Witczak, J. M. Andersen, S. Hicks, H. L. Hammer, E. Delbarre, P. Halvorsen, A. Yazidi, N. Holst and T. B. Haugen
Artificial intelligence in the fertility clinic: status, pitfalls and possibilities
Human Reproduction
A. Kolevatova, M. Riegler, F. Cherubini, X. Hu and H. L. Hammer
Unraveling the Impact of Land Cover Changes on Climate using Machine Learning and Explainable Artificial Intelligence
Big Data and Cognitive Computing
H. L. Hammer, A. Yazidi and H. Rue
Joint tracking of multiple quantiles through conditional quantiles
Information Sciences
P. H. Smedsrud, V. Thambawita, S. Hicks, H. Gjestang, O. O. Nedrejord, E. Næss, H. Borgli, D. Jha, T. J. D. Berstad, S. L. Eskeland, M. Lux, H. Espeland, A. Petlund, D. T. D. Nguyen, E. Garcia-Ceja, D. Johansen, P. T. Schmidt, E. Toth, H. L. Hammer, T. de Lange, M. Riegler and P. Halvorsen
Kvasir-Capsule, a video capsule endoscopy dataset
Scientific Data
A. A. Mofrad, A. Yazidi, S. A. Mofrad, H. L. Hammer and E. Arntzen
Enhanced Equivalence Projective Simulation: A Framework for Modeling Formation of Stimulus Equivalence Classes
Neural Computation
Books
M. Boeker, M. Riegler, H. L. Hammer, P. Halvorsen, O. B. Fasmer and P. Jakobsen
Diagnosing Schizophrenia from Activity Records using Hidden Markov Model Parameters
2021 IEEE 34th International Symposium on Computer-Based Medical Systems (CBMS)2021 IEEE 34th International Symposium on Computer-Based Medical Systems (CBMS)
Proceedings, refereed
S. Hicks, D. Jha, V. Thambawita, H. L. Hammer, T. de Lange, S. Parasa, M. Riegler and P. Halvorsen
Medico Multimedia Task at MediaEval 2021: Transparency in Medical Image Segmentation
Mediaeval Medico 2021
S. S. Eide, M. Riegler, H. L. Hammer and J. B. Bremnes
Temperature Forecasting using Tower Networks
Proceedings of the 2021 Workshop on Intelligent Cross-Data Analysis and Retrieval
S. Hicks, A. Stautland, O. B. Fasmer, W. Frøland, H. L. Hammer, P. Halvorsen, K. Mjeldheim, K. J. Oedegaard, B. Osnes, V. E. G. Syrstad, M. Riegler and P. Jakobsen
HYPERAKTIV: An Activity Dataset from Patients with Attention-Deficit/Hyperactivity Disorder (ADHD)
Proceedings of the 12th ACM Multimedia Systems Conference (MMSys '21)
V. Thambawita, T. B. Haugen, M. Stensen, O. Witczak, H. L. Hammer, P. Halvorsen and M. Riegler
Identification of spermatozoa by unsupervised learning from video data
37th Virtual Annual Meeting of the European Society of Human Reproduction and Embryology (ESHRE)
V. Thambawita, S. Hicks, J. Isaksen, M. H. Stensen, T. B. Haugen, J. Kanters, S. Parasa, T. de Lange, H. D. Johansen, D. Johansen, H. L. Hammer, P. Halvorsen and M. Riegler
DeepSynthBody: the beginning of the end for data deficiency in medicine
The International Conference on Applied Artificial Intelligence (ICAPAI)
E. Garcia-Ceja, V. Thambawita, S. Hicks, D. Jha, P. Jakobsen, H. L. Hammer, P. Halvorsen and M. Riegler
HTAD: A Home-Tasks Activities Dataset with Wrist-accelerometer and Audio Features
27th International Conference on Multimedia Modeling
2020
Journal Articles
A. A. Mofrad, A. Yazidi, H. L. Hammer and E. Arntzen
Equivalence projective simulation as a framework for modeling formation of stimulus equivalence classes
Neural computation
A. A. Mofrad, A. Yazidi, H. L. Hammer and E. Arntzen
Equivalence projective simulation as a framework for modeling formation of stimulus equivalence classes
Neural computation
A. Yazidi, H. L. Hammer, K. Samouylov and E. Herrera-Viedma
Game-Theoretic Learning for Sensor Reliability Evaluation Without Knowledge of the Ground Truth
IEEE Transactions on Cybernetics
A. Yazidi, M. A. Pinto-Orellana, H. L. Hammer, P. Mirtaheri and E. Herrera-Viedma
Solving Sensor Identification Problem Without Knowledge of the Ground Truth Using Replicator Dynamics
IEEE Transactions on Cybernetics
M. A. Pinto-Orellana and H. L. Hammer
Dyadic Aggregated Autoregressive Model (DASAR) for Automatic Modulation Classification
IEEE Access
A. Yazidi, A. A. Mofrad, M. Goodwin, H. L. Hammer and E. Arntzen
Balanced difficulty task finder: an adaptive recommendation method for learning tasks based on the concept of state of flow
Cognitive Neurodynamics
A. Yazidi, I. Hassan, H. L. Hammer and J. Oommen
Achieving Fair Load Balancing by Invoking a Learning Automata-Based Two-Time-Scale Separation Paradigm
IEEE Transactions on Neural Networks and Learning Systems