Data Science and Knowledge Discovery
The Department of Data Science and Knowledge Discovery (DataSci) aims to advance the frontiers of machine learning and data mining by developing novel methods and algorithms to analyse complex data sets and reveal underlying patterns. In doing so, we provide data mining methods that will enhance knowledge discovery in real-world applications in a range of fields – in particular, biomedicine.
Focus areas
Researchers at DataSci develop novel data mining/machine learning methods to analyse heterogeneous data sets collected from complex systems to reveal interpretable patterns that can help us better understand these systems.
Heterogeneous data sets are challenging to analyse since they are often multimodal (i.e., collected from multiple sources), incomplete data sets with missing entries and consist of both static and time-evolving data sets, in the form of multiway data with more than two axes of variation. Our research activities, in particular, focus on developing data fusion methods that can jointly analyse such heterogeneous data sets with the goal of revealing insights.
To understand complex systems like the brain, the metabolome, or at a larger scale, society, we need not only large amounts of data but also a means of making sense of it. In DataSci, our goal is to discover the hidden patterns in heterogeneous data to better understand the world around us.
Evrim Acar Ataman, Head of DataSci.
Research activities at DataSci span low-rank approximations (matrix/tensor factorizations), multimodal data mining (data fusion, coupled matrix/tensor factorisations), time series analysis, numerical linear algebra, multilinear algebra, and numerical optimisation. Researchers at DataSci are involved in interdisciplinary projects and have expertise in omics data analysis, in particular, analysis of (time-resolved) metabolomics data; neuroimaging data analysis and fusion of neuroimaging signals from multiple modalities; and social network analysis.
Using data to improve precision health
We develop data mining methods that hold the promise to advance precision health – one of our areas of interest is processes within the human brain and metabolome. We focus on developing unsupervised methods to reveal patterns from heterogeneous data sets, which can help us discover novel patient/subject stratifications, improve our understanding of underlying metabolic processes and reveal static/dynamic biomarkers of health and diseases.
One of our core projects is TrACEr (Time-aware Constrained Data Fusion), that develops data fusion methods to jointly analyse static and dynamic data sets with the goal of better understanding the human metabolome, how individuals differ in terms of their response to a meal.
Selected projects
Key partners
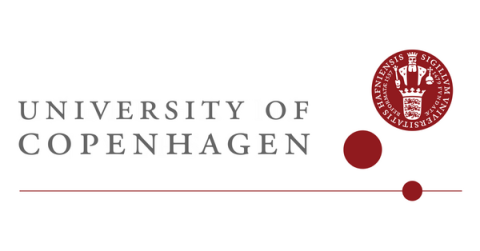
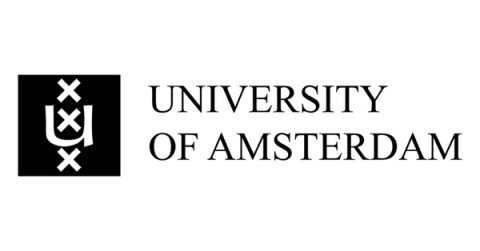
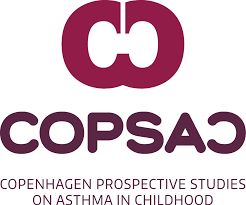
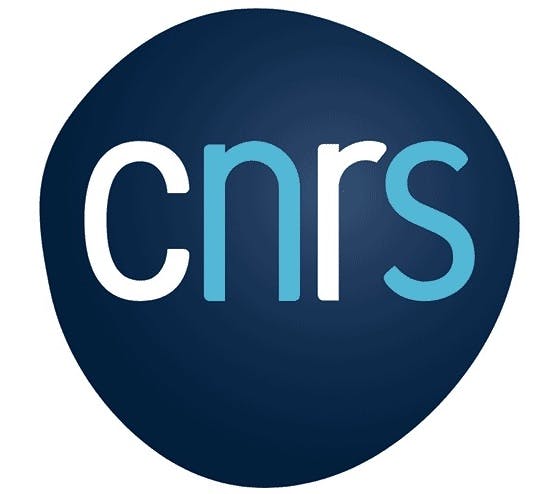
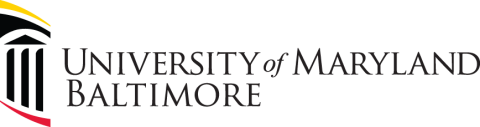