Signal and Information Processing for Intelligent Systems
SIGIPRO delivers innovative solutions for intelligent and multimodal sensor networks, information systems, and networked cyber-physical systems, by creating theories and algorithms that blend different disciplines. Our researchers work to establish synergies between fundamental theory, algorithmic solutions and application-specific implementations.
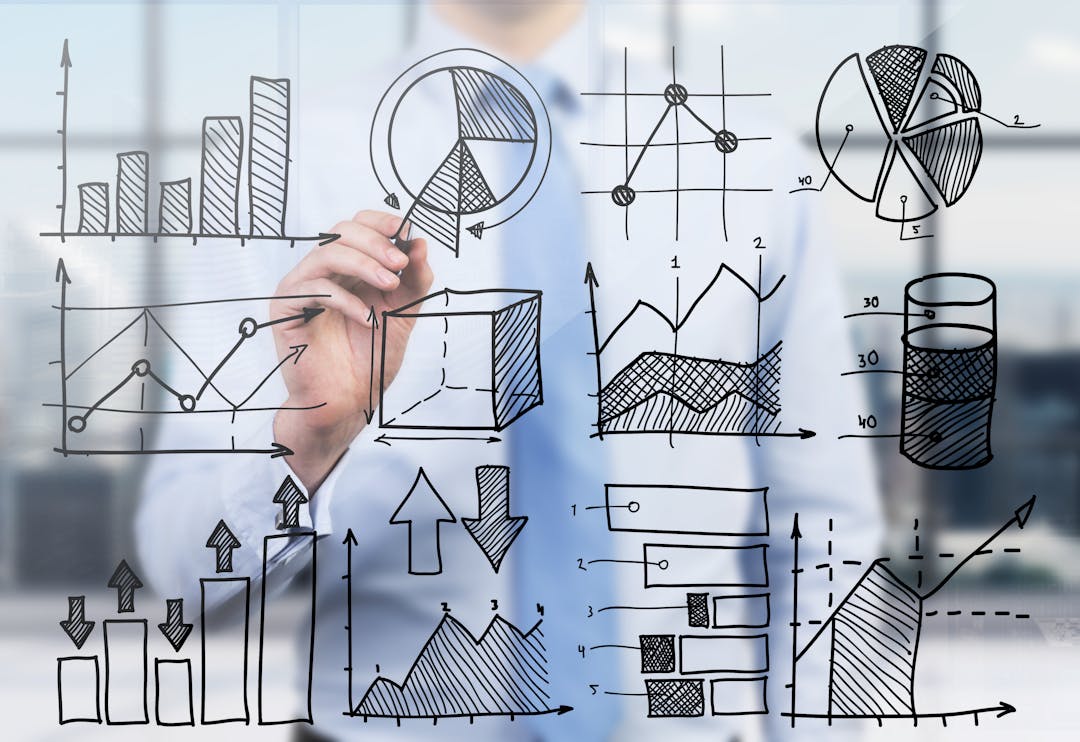
Focus areas
Intelligent systems acquire multimodal data from the environment, from which key information can be extracted. Based on this data, the system can learn rich models to describe the observed process (possibly combining both first principles and real data). Finally, the system can provide decision support to human operators or end-users, as well as make decisions directly or act autonomously to achieve a desired outcome. These systems can be either partially or totally decentralised and can have full or partial autonomy. Our solutions are specifically tailored to address energy efficiency and computational complexity requirements.
Our cross-disciplinary research approach combines tools and methods from signal processing, data science, machine learning, distributed intelligence, graph theory, numerical optimisation, wireless communications, autonomous multi-agent systems, complexity analysis, and decision control theory.
We are proud of our vibrant interdisciplinary work environment that supports talented researchers to develop innovative solutions that benefit society.
- Baltasar Beferull Lozano, Head of SIGIPRO
We investigate theoretical prescriptions that are used to guide the design of optimal, or close-to-optimal, algorithms, which give performance guarantees when possible, so that any heuristics are properly justified. These algorithms are tested both via simulation and using real testbed systems.
Ultimately, our goal is to deliver technical contributions that will benefit society. We aim to generate impact in many application domains, including Industry 4.0/5.0, digitalisation of process industry, smart water, energy and communication networks, e-health, brain signal processing for neuroscience, robotic avatars, collaborative robots, autonomous self-navigation, smart active sensing, human-machine interfaces, augmented reality, complex finance engineering systems, embedded intelligence for next-generation batteries, optimisation of processes in wind farms and hydropower plants, transportation, and biological networks.
Our impact
- SIGIPRO performs both theoretical and applied research that has an international impact, targeting the best international journals and conferences.
- We have strong national and international relationships through our projects and networks. Some of our closest collaborators include the Research Council of Norway, the Horizon Europe, and the EU European Research Council (ERC).
- SIGIPRO actively collaborates with private companies in developing applied research and has strong links with the private sector (technology, industry, finance) for technology transfer, patents, and creation of start-ups.
- We provide advanced academic training and guidance for PhD and master's students and recent graduates. We equip young professionals with the skills to conduct high quality research and to thrive in prestigious academic and high-tech innovation environments, both within Norway and internationally.